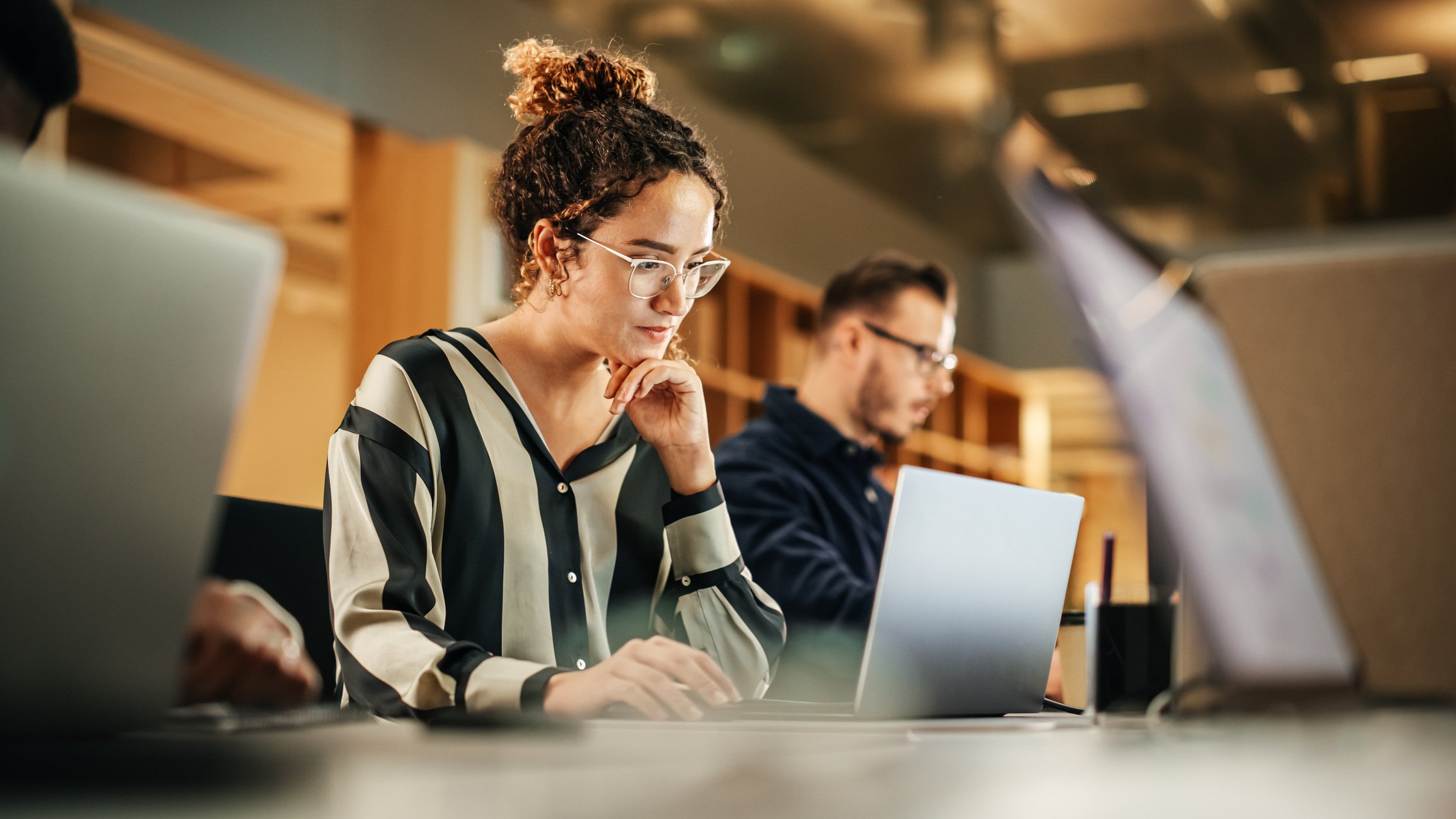
How Generative AI in Banking is Transforming Financial Services?
31 Oct, 2024, 3 min read
The banking industry is undergoing a shift as emerging technologies like generative AI reshape the landscape of financial services. While Need help deployingautomation and machine learning have already transformed banks' operations, generative AI brings a new level of sophistication to fraud detection and risk management. With cyber threats increasing in frequency and complexity, traditional methods are no longer sufficient. Banks need advanced tools to anticipate, prevent, and mitigate risks in real-time.
As banks have digitized their solutions with IT managed service providers, they have also become more vulnerable to fraud. Cybercriminals constantly refine their techniques, making it harder for traditional security measures to keep pace. From identity theft to phishing and account takeover, fraud has become a multi-faceted threat that costs the banking industry billions annually. The increased sophistication of fraud schemes makes it essential for banks to adopt more advanced technologies that can detect and respond to threats as they happen.
How Generative AI Enhances Fraud Detection
Fraud detection traditionally relies on identifying deviations from normal transactional behavior. However, this method often results in false positives, frustrating legitimate customers and slowing down banking operations. GenAI, on the other hand, offers a more nuanced approach by generating realistic models of user behavior, which can help distinguish between genuine and fraudulent activities more accurately.
- Real-Time Pattern Recognition:
Generative AI excels in recognizing patterns that humans or conventional systems might miss. By analyzing vast amounts of historical data, the AI can identify subtle changes in transaction behavior that could indicate fraud. These models are constantly updated with new data, enabling them to learn and adapt as new types of fraud emerge. For example, AI can recognize if a customer’s purchasing behavior suddenly shifts to locations or items that don’t align with their profile, signaling potential fraud.
- Dynamic Fraud Detection Models:
GenAI's ability to create synthetic datasets helps it simulate new types of fraud that have yet to be observed in the wild. This proactive approach gives banks a significant advantage, as they can detect previously unknown fraud patterns before they become widespread. Moreover, generative AI can develop multiple fraud detection models based on different scenarios, offering a layered approach to identifying complex fraudulent schemes.
- Reducing False Positives:
One of the major pain points in fraud detection is the high rate of false positives, where legitimate transactions are flagged as fraudulent. Generative AI’s advanced behavioral analysis can more accurately predict which transactions are fraudulent, leading to fewer false alarms. This reduces friction for customers while improving operational efficiency for banks.
Generative AI and Risk Management
Risk management in banking is a broad discipline that encompasses credit, operational, and market risks. Generative AI enhances risk management by offering predictive insights, advanced simulation capabilities, and more accurate risk modeling.
- Predictive Risk Modeling:
Gen AI can build more accurate risk models by analyzing vast amounts of structured and unstructured data, including market trends, customer behavior, and even external factors like geopolitical events. By predicting potential risk factors, banks can make more informed decisions on lending, investment strategies, and operational planning. For example, generative AI can forecast potential credit risk by simulating how a customer might respond to changing economic conditions, helping banks adjust loan terms or approve new applicants more confidently.
- Stress Testing and Scenario Planning:
In traditional risk management, stress testing involves running hypothetical scenarios to see how a bank would respond under adverse conditions, such as an economic downturn or a market crash. Generative AI enhances this process by creating more sophisticated simulations, offering a broader range of scenarios that could impact the bank. This not only improves the accuracy of stress testing but also provides a clearer picture of potential vulnerabilities within the organization.
- Real-Time Risk Assessment:
Generative AI can offer real-time insights into a bank’s risk exposure, allowing decision-makers to respond immediately to emerging risks. By continuously monitoring market trends and customer behavior, generative AI can alert banks to potential issues before they escalate. This is particularly useful in high-frequency trading environments, where a few seconds delay can result in significant financial losses.
Need help deploying AI Solutions at your organization? Contact the Atlas experts today at sales@atlassystems.com to schedule a discussion about your AI requirements.
stay ahead of CMS deadlines!